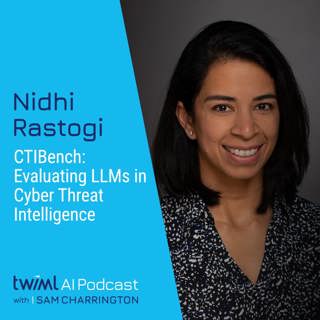
CTIBench: Evaluating LLMs in Cyber Threat Intelligence with Nidhi Rastogi - #729
Today, we're joined by Nidhi Rastogi, assistant professor at Rochester Institute of Technology to discuss Cyber Threat Intelligence (CTI), focusing on her recent project CTIBench—a benchmark for evaluating LLMs on real-world CTI tasks. Nidhi explains the evolution of AI in cybersecurity, from rule-based systems to LLMs that accelerate analysis by providing critical context for threat detection and defense. We dig into the advantages and challenges of using LLMs in CTI, how techniques like Retrieval-Augmented Generation (RAG) are essential for keeping LLMs up-to-date with emerging threats, and how CTIBench measures LLMs’ ability to perform a set of real-world tasks of the cybersecurity analyst. We unpack the process of building the benchmark, the tasks it covers, and key findings from benchmarking various LLMs. Finally, Nidhi shares the importance of benchmarks in exposing model limitations and blind spots, the challenges of large-scale benchmarking, and the future directions of her AI4Sec Research Lab, including developing reliable mitigation techniques, monitoring "concept drift" in threat detection models, improving explainability in cybersecurity, and more. The complete show notes for this episode can be found at https://twimlai.com/go/729.
30 Apr 56min
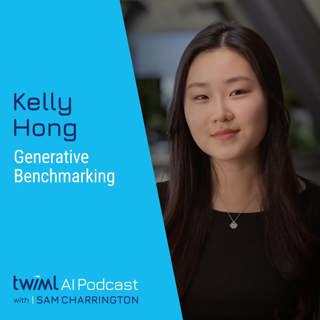
Generative Benchmarking with Kelly Hong - #728
In this episode, Kelly Hong, a researcher at Chroma, joins us to discuss "Generative Benchmarking," a novel approach to evaluating retrieval systems, like RAG applications, using synthetic data. Kelly explains how traditional benchmarks like MTEB fail to represent real-world query patterns and how embedding models that perform well on public benchmarks often underperform in production. The conversation explores the two-step process of Generative Benchmarking: filtering documents to focus on relevant content and generating queries that mimic actual user behavior. Kelly shares insights from applying this approach to Weights & Biases' technical support bot, revealing how domain-specific evaluation provides more accurate assessments of embedding model performance. We also discuss the importance of aligning LLM judges with human preferences, the impact of chunking strategies on retrieval effectiveness, and how production queries differ from benchmark queries in ambiguity and style. Throughout the episode, Kelly emphasizes the need for systematic evaluation approaches that go beyond "vibe checks" to help developers build more effective RAG applications. The complete show notes for this episode can be found at https://twimlai.com/go/728.
23 Apr 54min
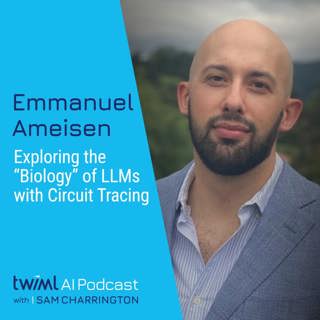
Exploring the Biology of LLMs with Circuit Tracing with Emmanuel Ameisen - #727
In this episode, Emmanuel Ameisen, a research engineer at Anthropic, returns to discuss two recent papers: "Circuit Tracing: Revealing Language Model Computational Graphs" and "On the Biology of a Large Language Model." Emmanuel explains how his team developed mechanistic interpretability methods to understand the internal workings of Claude by replacing dense neural network components with sparse, interpretable alternatives. The conversation explores several fascinating discoveries about large language models, including how they plan ahead when writing poetry (selecting the rhyming word "rabbit" before crafting the sentence leading to it), perform mathematical calculations using unique algorithms, and process concepts across multiple languages using shared neural representations. Emmanuel details how the team can intervene in model behavior by manipulating specific neural pathways, revealing how concepts are distributed throughout the network's MLPs and attention mechanisms. The discussion highlights both capabilities and limitations of LLMs, showing how hallucinations occur through separate recognition and recall circuits, and demonstrates why chain-of-thought explanations aren't always faithful representations of the model's actual reasoning. This research ultimately supports Anthropic's safety strategy by providing a deeper understanding of how these AI systems actually work. The complete show notes for this episode can be found at https://twimlai.com/go/727.
14 Apr 1h 34min
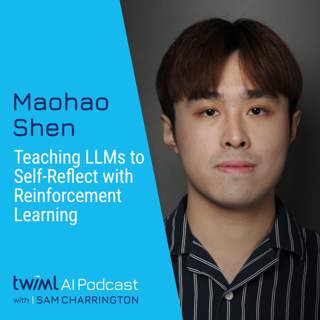
Teaching LLMs to Self-Reflect with Reinforcement Learning with Maohao Shen - #726
Today, we're joined by Maohao Shen, PhD student at MIT to discuss his paper, “Satori: Reinforcement Learning with Chain-of-Action-Thought Enhances LLM Reasoning via Autoregressive Search.” We dig into how Satori leverages reinforcement learning to improve language model reasoning—enabling model self-reflection, self-correction, and exploration of alternative solutions. We explore the Chain-of-Action-Thought (COAT) approach, which uses special tokens—continue, reflect, and explore—to guide the model through distinct reasoning actions, allowing it to navigate complex reasoning tasks without external supervision. We also break down Satori’s two-stage training process: format tuning, which teaches the model to understand and utilize the special action tokens, and reinforcement learning, which optimizes reasoning through trial-and-error self-improvement. We cover key techniques such “restart and explore,” which allows the model to self-correct and generalize beyond its training domain. Finally, Maohao reviews Satori’s performance and how it compares to other models, the reward design, the benchmarks used, and the surprising observations made during the research. The complete show notes for this episode can be found at https://twimlai.com/go/726.
8 Apr 51min
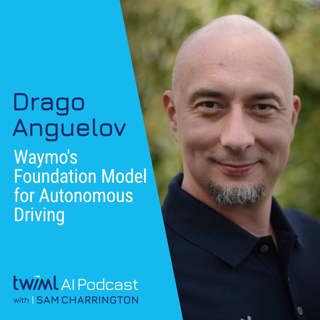
Waymo's Foundation Model for Autonomous Driving with Drago Anguelov - #725
Today, we're joined by Drago Anguelov, head of AI foundations at Waymo, for a deep dive into the role of foundation models in autonomous driving. Drago shares how Waymo is leveraging large-scale machine learning, including vision-language models and generative AI techniques to improve perception, planning, and simulation for its self-driving vehicles. The conversation explores the evolution of Waymo’s research stack, their custom “Waymo Foundation Model,” and how they’re incorporating multimodal sensor data like lidar, radar, and camera into advanced AI systems. Drago also discusses how Waymo ensures safety at scale with rigorous validation frameworks, predictive world models, and realistic simulation environments. Finally, we touch on the challenges of generalization across cities, freeway driving, end-to-end learning vs. modular architectures, and the future of AV testing through ML-powered simulation. The complete show notes for this episode can be found at https://twimlai.com/go/725.
31 Mars 1h 9min
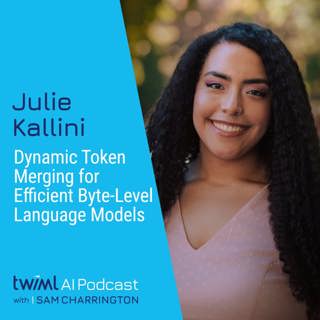
Dynamic Token Merging for Efficient Byte-level Language Models with Julie Kallini - #724
Today, we're joined by Julie Kallini, PhD student at Stanford University to discuss her recent papers, “MrT5: Dynamic Token Merging for Efficient Byte-level Language Models” and “Mission: Impossible Language Models.” For the MrT5 paper, we explore the importance and failings of tokenization in large language models—including inefficient compression rates for under-resourced languages—and dig into byte-level modeling as an alternative. We discuss the architecture of MrT5, its ability to learn language-specific compression rates, its performance on multilingual benchmarks and character-level manipulation tasks, and its performance and efficiency. For the “Mission: Impossible Language Models” paper, we review the core idea behind the research, the definition and creation of impossible languages, the creation of impossible language training datasets, and explore the bias of language model architectures towards natural language. The complete show notes for this episode can be found at https://twimlai.com/go/724.
24 Mars 50min
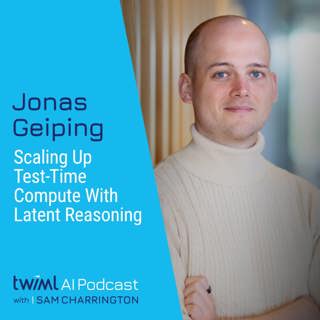
Scaling Up Test-Time Compute with Latent Reasoning with Jonas Geiping - #723
Today, we're joined by Jonas Geiping, research group leader at Ellis Institute and the Max Planck Institute for Intelligent Systems to discuss his recent paper, “Scaling up Test-Time Compute with Latent Reasoning: A Recurrent Depth Approach.” This paper proposes a novel language model architecture which uses recurrent depth to enable “thinking in latent space.” We dig into “internal reasoning” versus “verbalized reasoning”—analogous to non-verbalized and verbalized thinking in humans, and discuss how the model searches in latent space to predict the next token and dynamically allocates more compute based on token difficulty. We also explore how the recurrent depth architecture simplifies LLMs, the parallels to diffusion models, the model's performance on reasoning tasks, the challenges of comparing models with varying compute budgets, and architectural advantages such as zero-shot adaptive exits and natural speculative decoding. The complete show notes for this episode can be found at https://twimlai.com/go/723.
17 Mars 58min
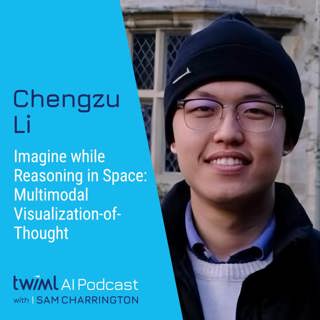
Imagine while Reasoning in Space: Multimodal Visualization-of-Thought with Chengzu Li - #722
Today, we're joined by Chengzu Li, PhD student at the University of Cambridge to discuss his recent paper, “Imagine while Reasoning in Space: Multimodal Visualization-of-Thought.” We explore the motivations behind MVoT, its connection to prior work like TopViewRS, and its relation to cognitive science principles such as dual coding theory. We dig into the MVoT framework along with its various task environments—maze, mini-behavior, and frozen lake. We explore token discrepancy loss, a technique designed to align language and visual embeddings, ensuring accurate and meaningful visual representations. Additionally, we cover the data collection and training process, reasoning over relative spatial relations between different entities, and dynamic spatial reasoning. Lastly, Chengzu shares insights from experiments with MVoT, focusing on the lessons learned and the potential for applying these models in real-world scenarios like robotics and architectural design. The complete show notes for this episode can be found at https://twimlai.com/go/722.
10 Mars 42min